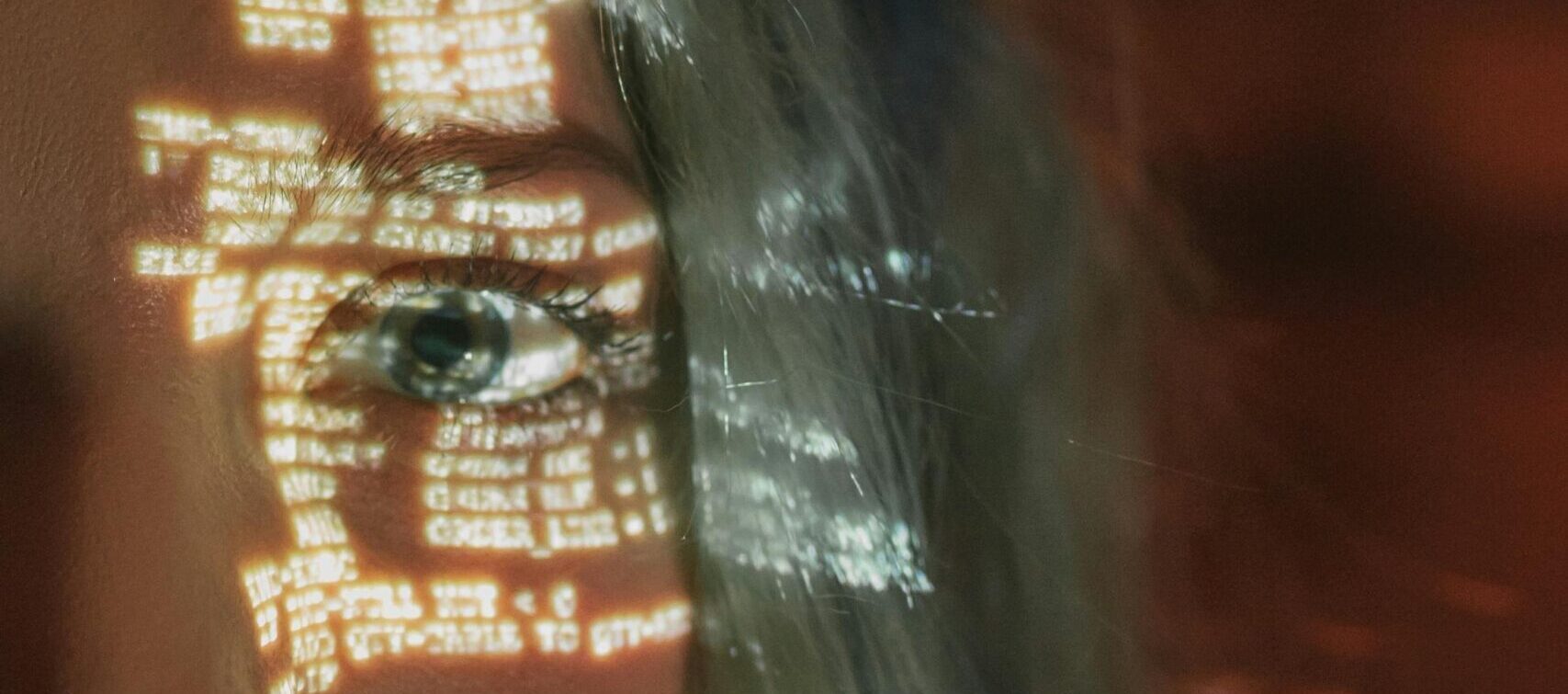
Aug
Can Synthetic Respondents Take Over Surveys?
jerry97890 comments artificial intelligence, Burning Questions
What Are Synthetic Respondents?
AI has increased operational efficiency by streamlining knowledge bases and shortcutting processes so it’s no surprise people and companies are looking for more ways for its application. For market research, one curious consideration is whether it could take over surveys, essentially by replacing actual respondents with synthetic respondents.
Also known as virtual respondents, digital personas, and Virtual Audiences, synthetic respondents are individual profiles constructed by Large Language Models (LLMs) from real or simulated data. Ideally, the data or descriptions used to generate these profiles come from previously conducted surveys and are combined with individual-level demographics, attitudes and behaviors.
Using these synthetic respondents over real respondents could benefit your research with speed, accuracy and cost savings, at least according to their advocates. Basically, you just need to conduct one survey and from the profile description or data you gathered from the actual respondents, you’re able to generate results from the constructed individuals over and over for succeeding studies and research.
Testing Synthetic Respondents
While synthetic respondents could accurately represent real respondents, relying exclusively on the results from these AI-based individuals may not be entirely beneficial. A webinar hosted by Radius Global took a closer look at the potential of AI-generated synthetic respondents through three case studies of quantitative concept testing, quantitative communications research, and qualitative communications research.
Aggregate results for the concept tests involving game controllers indicate somewhat strong similarities between the results of the real and synthetic respondents. This extends to the results from the quantitative communications research when it comes to the believability of statements on the benefits of milk, although there were some differences. The differences were much more pronounced though when it comes to surprise over the same statements, and there was incongruence when considering how each statement could possibly increase milk consumption.
The qualitative communications research was seeking in-depth insights into women’s needs, perceptions, and preferences for running a race or marathon, with the feedback gathered meant to be used for creative content. Personas were constructed from the profiles of six women aged between 18 and 64 years old who ran at least once in an average week. They had an LLM assume each persona to allow a comparison between findings from real participants to synthetic respondents.
They found that while both real and synthetic respondents have somewhat similar responses when it comes to functional aspects as goals for women in general pursuing fitness, the AI responses lacked emotional expressions. There are also little differences in the synthetic respondents’s responses despite having different profiles, and there was even a lack of subtle differences.
As for concerns among women who are aspirational marathon runners, the synthetic personas were consistent in their responses while the real respondents provided more nuances, variety, and perspectives more prevalent among women.
Synthetic Respondents Vs. Real Respondents
Synthetic respondents appear to be useful if you’re evaluating existing ideas and concepts; however, if you’re looking for “breakthroughs” or essentially new insights you would’ve never arrived at had you not performed the case study or research, you would need to engage with real respondents, relying exclusively on their results or combining them with that of synthetic respondents. Yes, there could be cases where synthetic respondents could be used, but the results must be extensively validated. It would also require increasing the efficiency of how data used to construct these individuals are analyzed in addition to enhancing the quality of the data and information gathered for these profiles through thorough screening, intelligent probing, and smart choice models.
There is a place for synthetic respondents in market research, but as another tool in a researcher’s disposal. They won’t be taking over surveys nor replacing actual respondents wholesale anytime soon it seems, as that elusive “Eureka” moment researchers seek are inherently tied with the nuances and perspectives of human emotion and experience you just simply couldn’t construct.