Showing posts tagged with: ai revolution
24
Apr
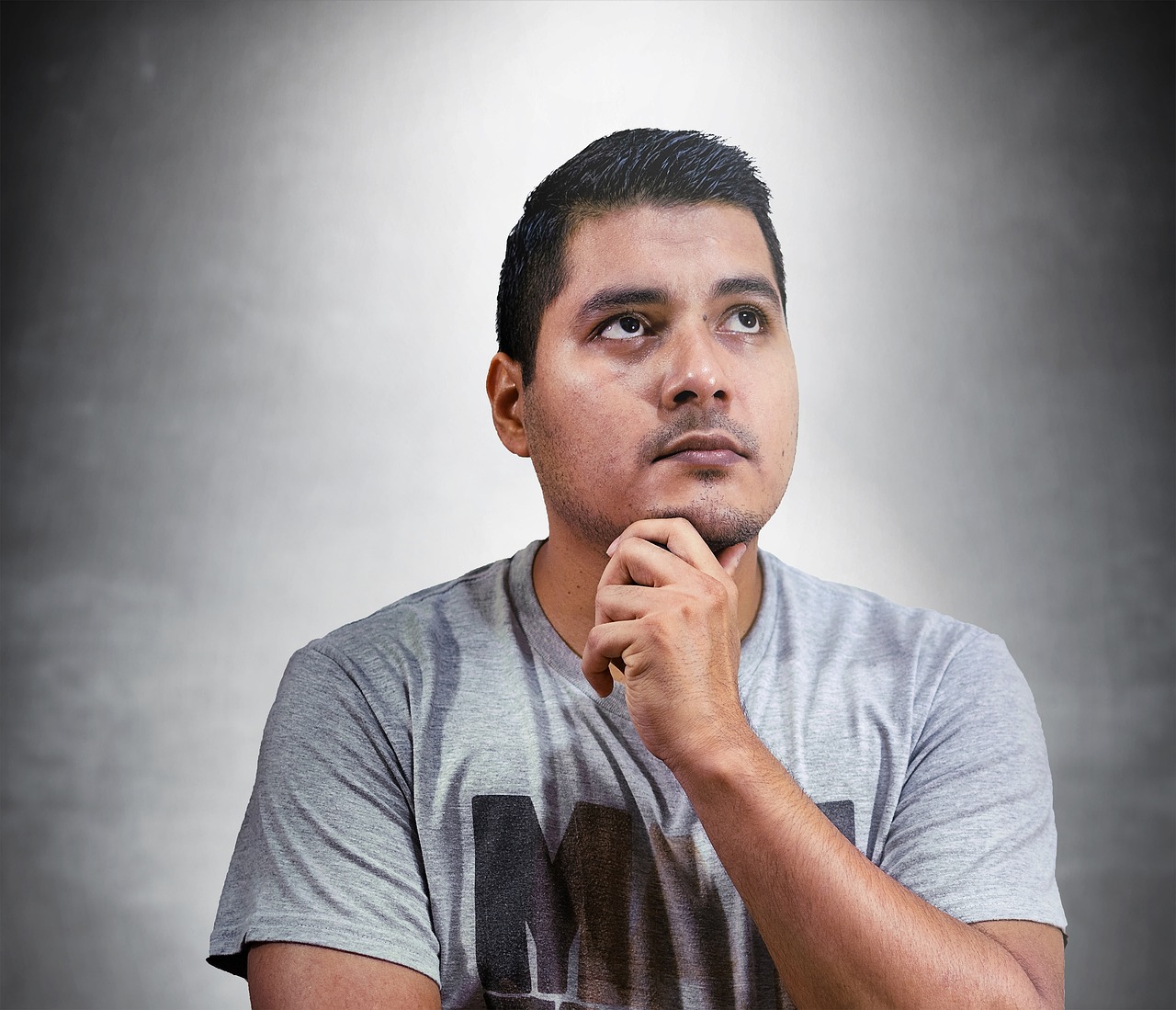
Generative AI and Critical Thinking
On our last blog, we touched on two studies suggesting that Generative AI is making us dumber. One of those studies, which was published in the journal Societies, aimed to look deeper into GenAI’s impact on our critical thinking by surveying and interviewing over 600 UK participants of varying age groups and academic backgrounds. The study found “a significant negative correlation between frequent AI tool usage and critical thinking abilities, mediated by increased cognitive offloading.”
Cognitive offloading refers to the utilization of external tools and processes to simplify tasks or optimize productivity. Cognitive offloading has always raised concerns over the perceived decline of certain skills — in this instance, the dulling of one’s critical thinking. In fact, the study found that cognitive offloading was worse with younger participants who demonstrated higher reliance on AI tools and less aptitude when it comes to their own critical thinking skills.
Conversely, participants with higher educational backgrounds showed better command of their critical thinking no matter the degree of AI usage, putting more confidence in their own mental acuity than the AI-based outputs. Aligning with our advocacy for the “appropriate use of AI,” the study emphasizes the importance and appreciation of high-level human thinking over thoughtless and unmitigated adoption of AI technology.
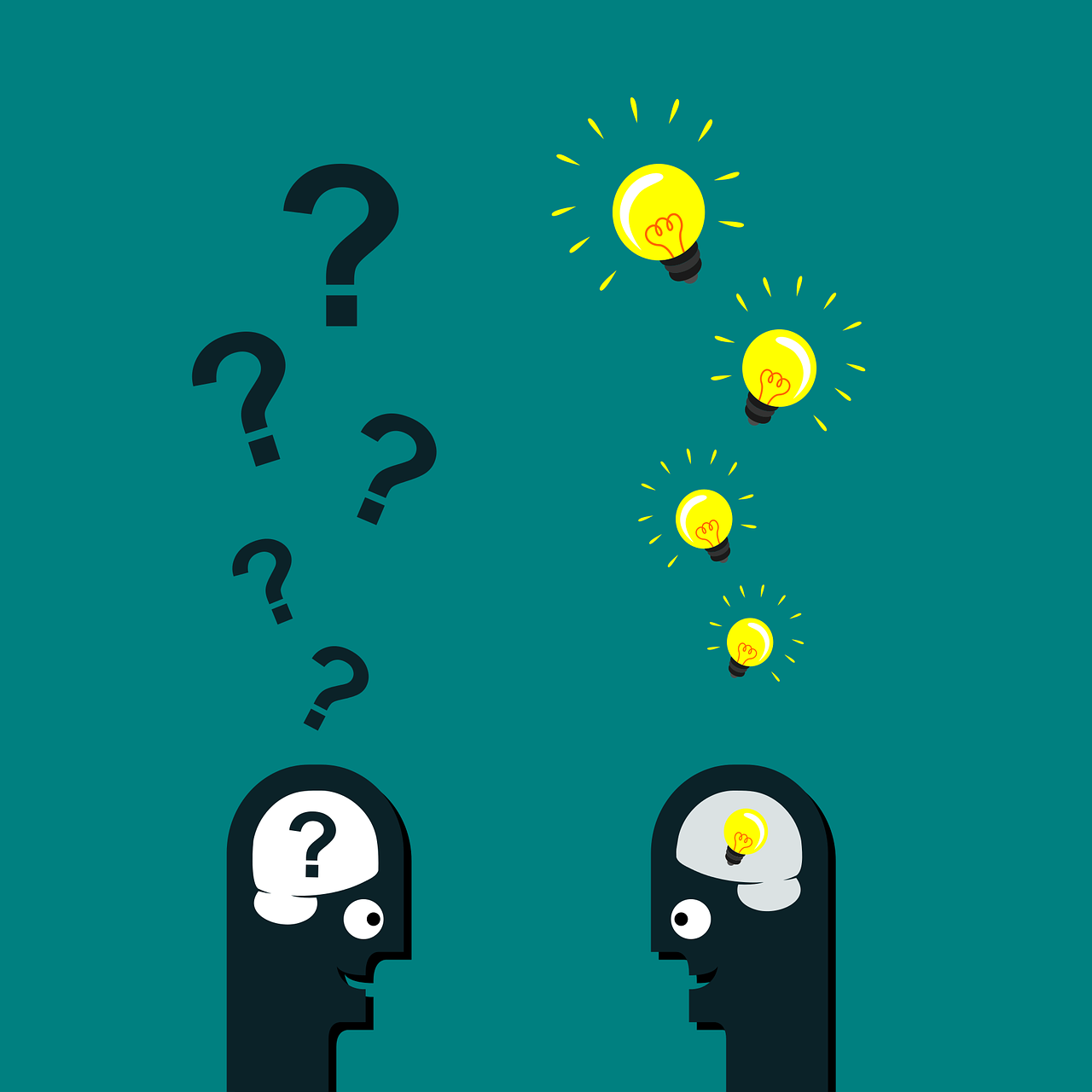
Copyright: jambulboy
Generative AI and Learning
In truth, a number of earlier studies have revealed that the arbitrary adoption of AI tools can be detrimental to one’s ability to learn or develop new skills. A 2024 Wharton study on the impact of OpenAI’s GPT-4 demonstrated that unmitigated deployment of GenAI fostered overreliance on the technology as a “crutch” and led to poor performance when such tools are taken away. The field experiment involved 1,000 high school math students who, following a math lesson, were asked to solve a practice test. They were divided into three groups, with two of these groups having access to ChatGPT while the third had only their class notes. One group of students with ChatGPT performed 48 percent better than those without; however, a follow-up exam without the aid of any laptop or books saw the same students scoring worse by 17 percent than their peers who had only their notes.
What about the second group with the GenAI tutor? They not only performed 127 percent higher than the group without ChatGPT access on the first exam, but they also scored close to the latter during the follow-up exam. The difference? Sometime down the line of their interactions, the first group with ChatGPT access would prompt their AI tutor to divulge the answers, resulting in an increased reliance on GenAI to provide the solutions instead of making use of their own problem-solving abilities. On the other hand, the other group’s AI tutor version was customized to be closer to how real-world and highly effective tutors would interact with students: it would help by giving hints and providing feedback on the learner’s performance, but it would never directly give the answer.
Similar tests with a GenAI tutor in 2023 studied the same issue of AI dependence and the value of careful deployment of AI tools. Khanmigo, a GenAI tutor developed by Khan Academy, was voluntarily tested by Newark elementary school teachers, who belong to the largest public school system in New Jersey. They came back with mixed results, with some complaining that the AI tutor gave away answers, even incorrect ones in some cases, while others appreciated the bot’s usefulness as a “co-teacher.”
Other studies regarding the effectiveness of AI tutors have shown increases in learning and student engagement. These studies have also shown that GenAI can help reduce the time it takes to get through learning materials compared to traditional methods. One study that extolled the benefits of GenAI tutors involved Harvard undergraduates learning physics in 2024, and similar to the third group in the Wharton research, the AI was prevented from directly providing the answer to students. It would guide the student throughout the learning process one step at a time, providing incremental updates of the student’s progress, but never outright telling them the answer. There are merits to the idea of Generative AI as a teaching assistant, but it serves students better when it is positioned to engage one’s attention and abilities rather than induce dependence on it to generate the answers.
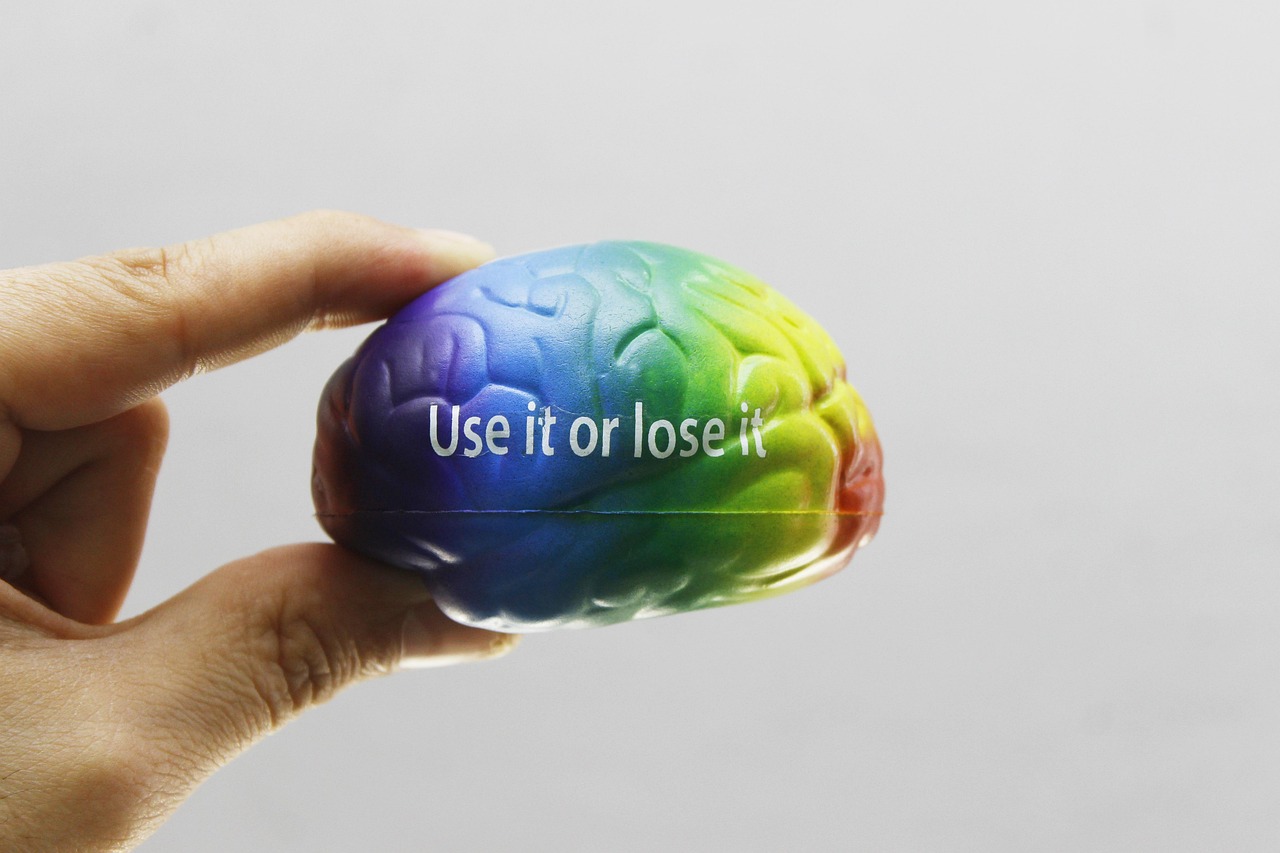
Copyright: Only-shot
Can We Use GenAI Without Making Us Dumber?
These studies shed light on how we should approach AI solutions and development, whether the end product is being deployed in learning, productivity or other relevant applications. Beyond thoughtful planning and considerations on how AI tools would be deployed, there should be a focus on engaging the human faculties involved, with safeguards empowering man throughout the entire process instead of letting the machine take over the process wholesale. AI technology is developing rapidly, but we can keep pace and remain reasonable as long as human engagement and empowerment is kept at the core of its utilization and adoption.
Amid contemporary fears that anyone could be replaced anytime by AI, these studies highlight the importance of how vital and interconnected the human factor is to the effective deployment and development of AI tools. One could be content with the constant and consistent output AI tools generate, but progress is only possible when competent human minds are involved in the process and direction. Students can easily find answers with AI tools at their disposal, but why not advance their understanding of how solutions are formed with engaging and relatable AI-powered educational experiences? High-level human thinking grounded by values and experience can’t be replicated by machines, and perhaps there’s no better time than now to incorporate it into the heart of the AI revolution.
While AI development hopes that optimization and automation free the human mind to go after bigger and more creative pursuits, we here at Cascade Strategies simply hope that humanity emerges from all of these advancements more and not less than what it as we entered the AI revolution.
Additional Reading:
Why AI is no substitute for human teachers – Megan Morrone, Axios
AI Tutors Can Work—With the Right Guardrails – Daniel Leonard, Edutopia
Featured Image Copyright: jallen_RTR
Top Image Copyright: danymena88
Read more
07
Feb
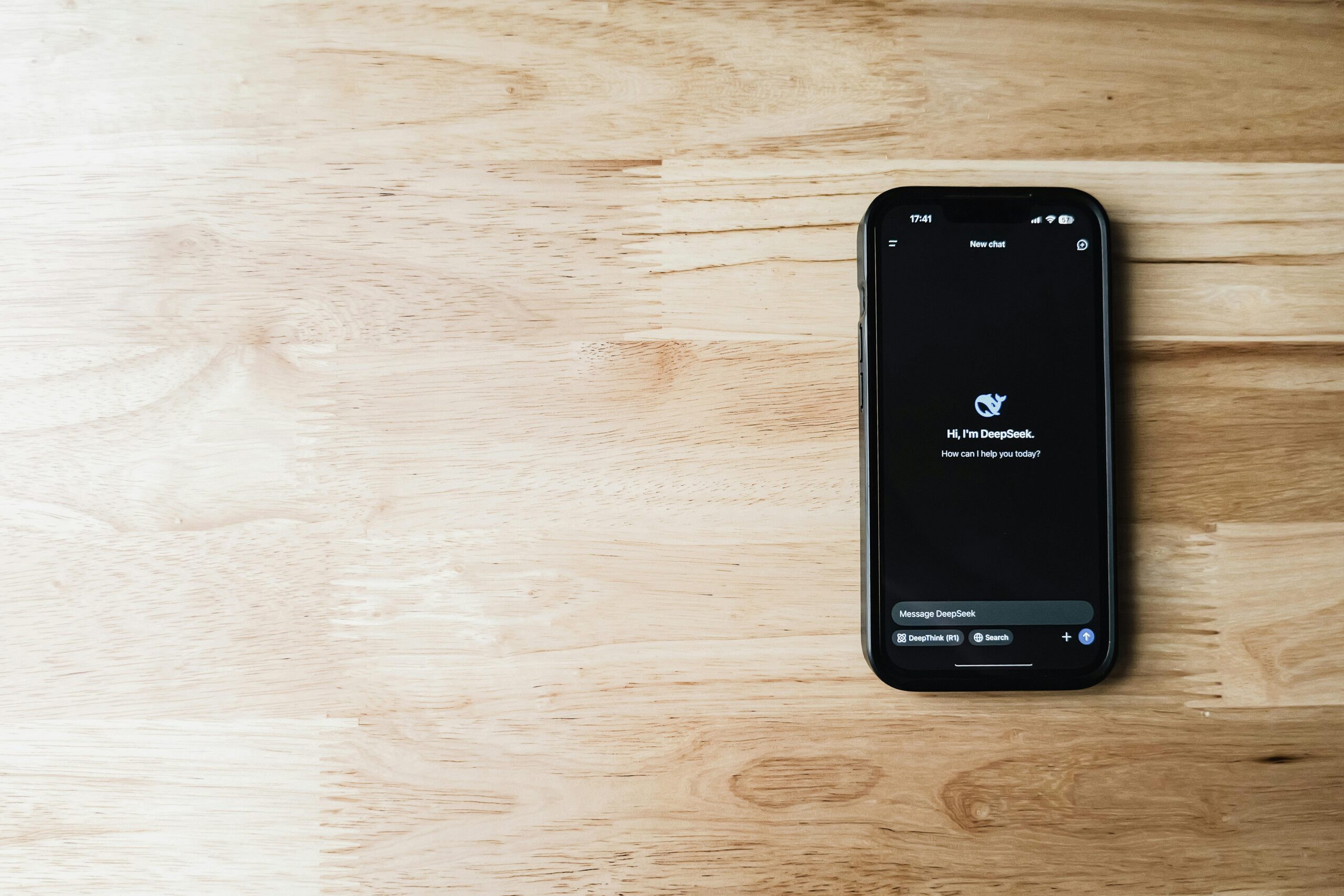
Paradigm Shift
We’ve recently written about recent AI advancements and popularity, particularly generative AI like that of ChatGPT, driving renewed demand for data centers not seen in decades. This surging demand pushed tech investors to put $39.6 billion into data center development in 2024, which is 12 times the amount invested back in 2016.
A recent development, however, has stirred things up, especially the concept that billions of dollars needed to be spent for AI advancement. Developed by a Chinese AI research lab, an open-source large language model named DeepSeek was released and performed on par with OpenAI, but it reportedly operates for just a fraction of the cost of Western AI models. OpenAI, however, is investigating if DeepSeek utilized distillation of the former’s AI models to develop their systems.
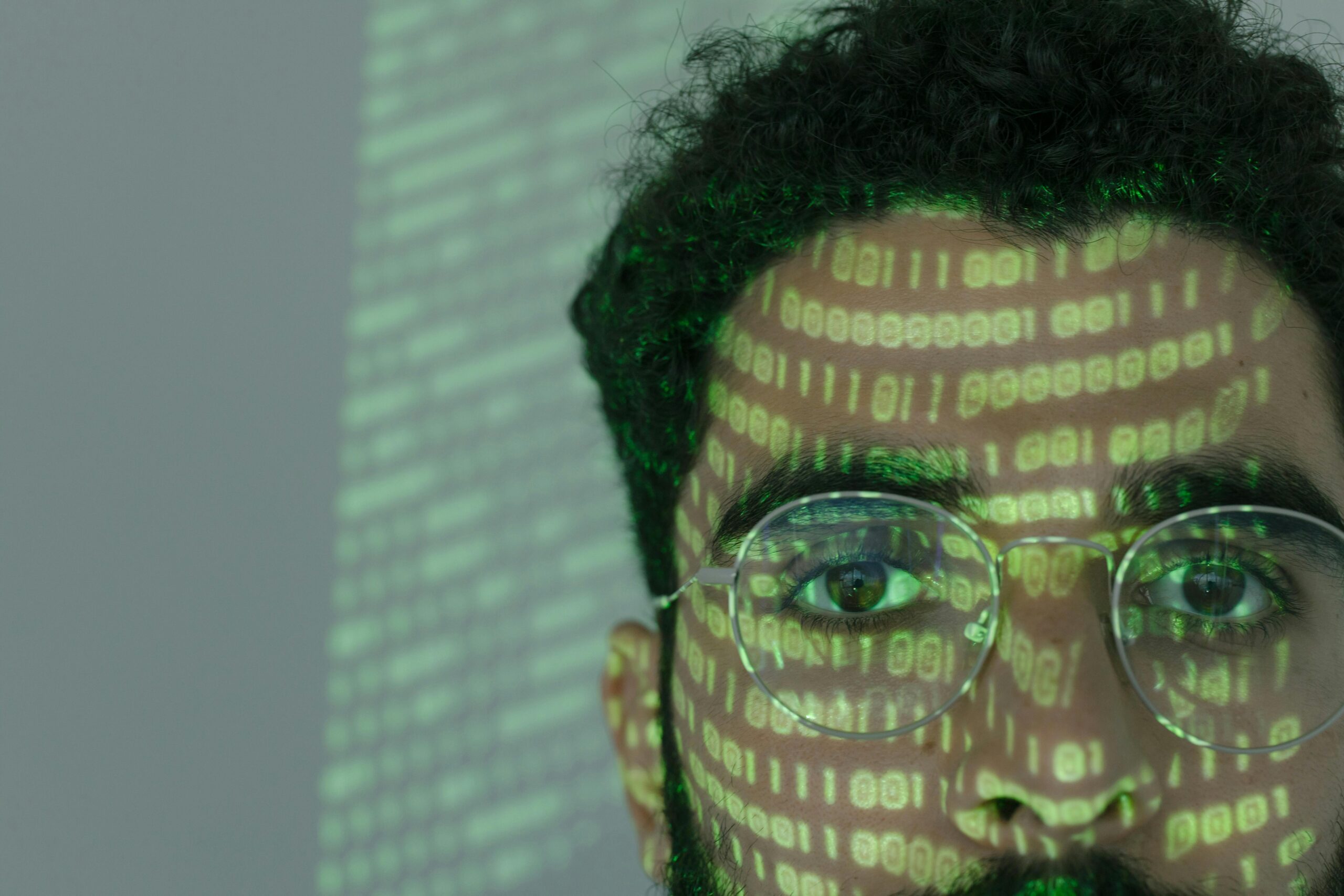
Copyright: cottonbro studio
What Is “Distillation?”
According to Labelbox, model distillation (or knowledge distillation) is a machine learning technique involving the transfer of knowledge from a large model to a smaller one. Distillation bridges the gap between computational demand and the cost for training large models while maintaining performance. Basically, the large model learns from an enormous amount of raw data for a number of months and a huge sum of money typically in a training lab, then passes on that knowledge to its smaller counterpart primed for real-world application and production for less time and money.
Distillation has been around for some time and has been used by AI developers, but not to the same degree of success as DeepSeek. The Chinese AI developer had said that aside from their own models, they also distilled from open-source AIs released by Meta Platforms and Alibaba.
However, the terms of service for OpenAI prohibits the use of its models for developing competing applications. While OpenAI had banned suspected accounts for distillation during its investigation, US President Donald Trump’s AI czar David Sacks had called out DeepSeek for distilling from OpenAI models. Sacks added that US AI companies should take measures to protect their models or make it difficult for their models to be distilled.
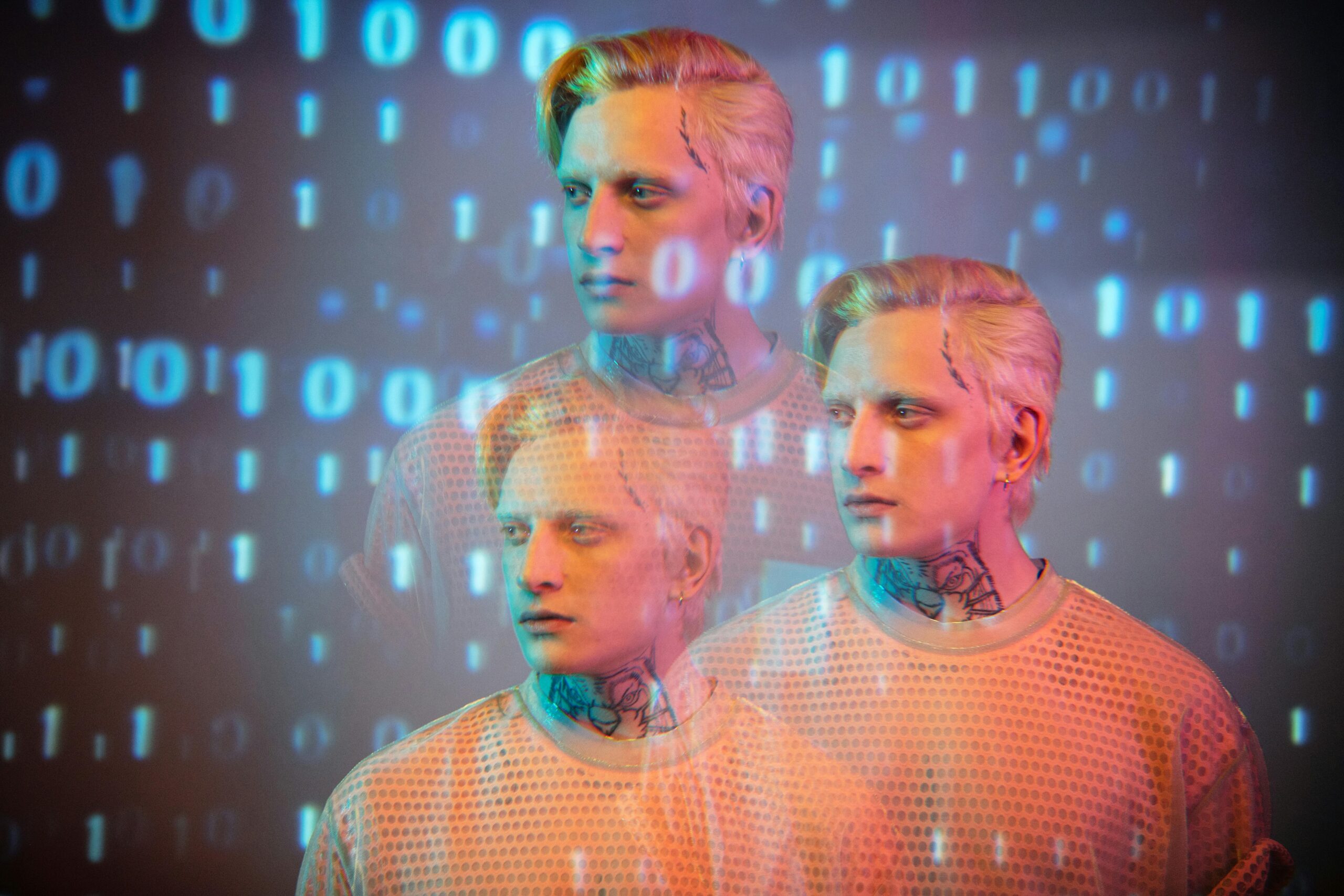
Copyright: Darlene Anderson
How Does Distillation Affect AI Investments?
On the back of DeepSeek’s success, distillation might give tech giants cause to reexamine their business models and investors to question the amount of dollars they put into AI advancements. Is it worth it to be a pioneer or industry leader when the same efforts can be replicated by smaller rivals at less cost? Can an advantage still exist for tech companies that ask for huge investments to blaze a trail when others are too quick to follow and build upon the leader’s achievements?
A recent Wall Street Journal article notes that tech executives expect distillation to produce more high-quality models. The same article mentions Anthropic CEO Dario Amodei blogging that DeepSeek’s R1 model “is not a unique breakthrough or something that fundamentally changes the economics” of advanced AI systems. This is an expected development as the costs for AI operations continue to fall and models move towards being more open-source.
Perhaps that’s where the advantage for tech leaders and investors lies: the opportunity to break new ground and the understanding that you’re seeking answers from unexplored spaces while the rest limit themselves and reiterate within the same technological confines. Established tech giants continue to enjoy the prestige of their AI models being more widely used in Silicon Valley — despite DeepSeek’s economical advantage — and the expectation of being the first to bring new advancements and developments to the digital world.
And maybe, just maybe, in that space between the pursuit of new AI breakthroughs and lower-cost AI models lie solutions to help meet the increasing demand for data centers and computing power.
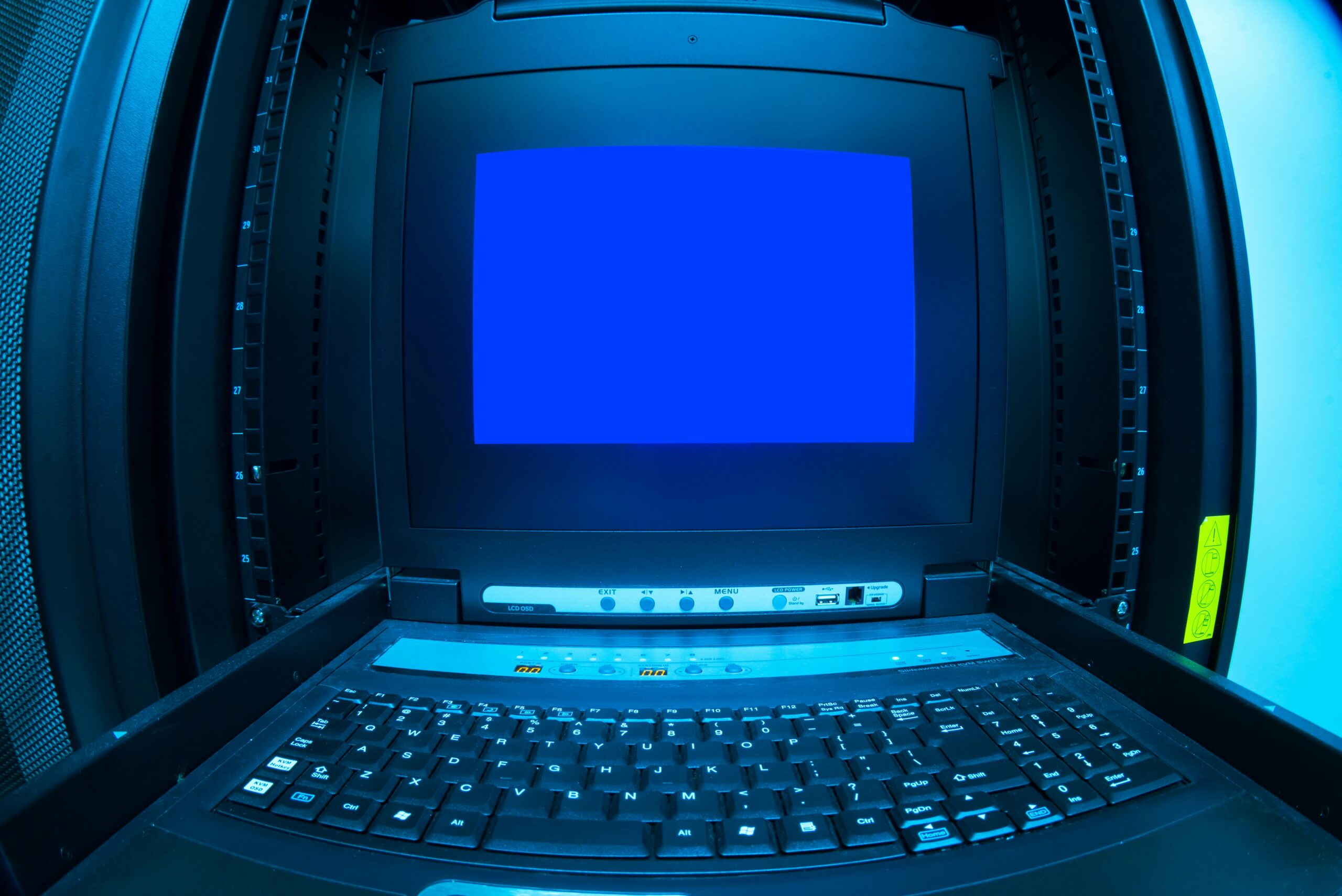
Copyright: panumas nikhomkhai
Featured Image Copyright: Matheus Bertelli
Top Image Copyright: Airam Dato-on
Read more
28
Jan
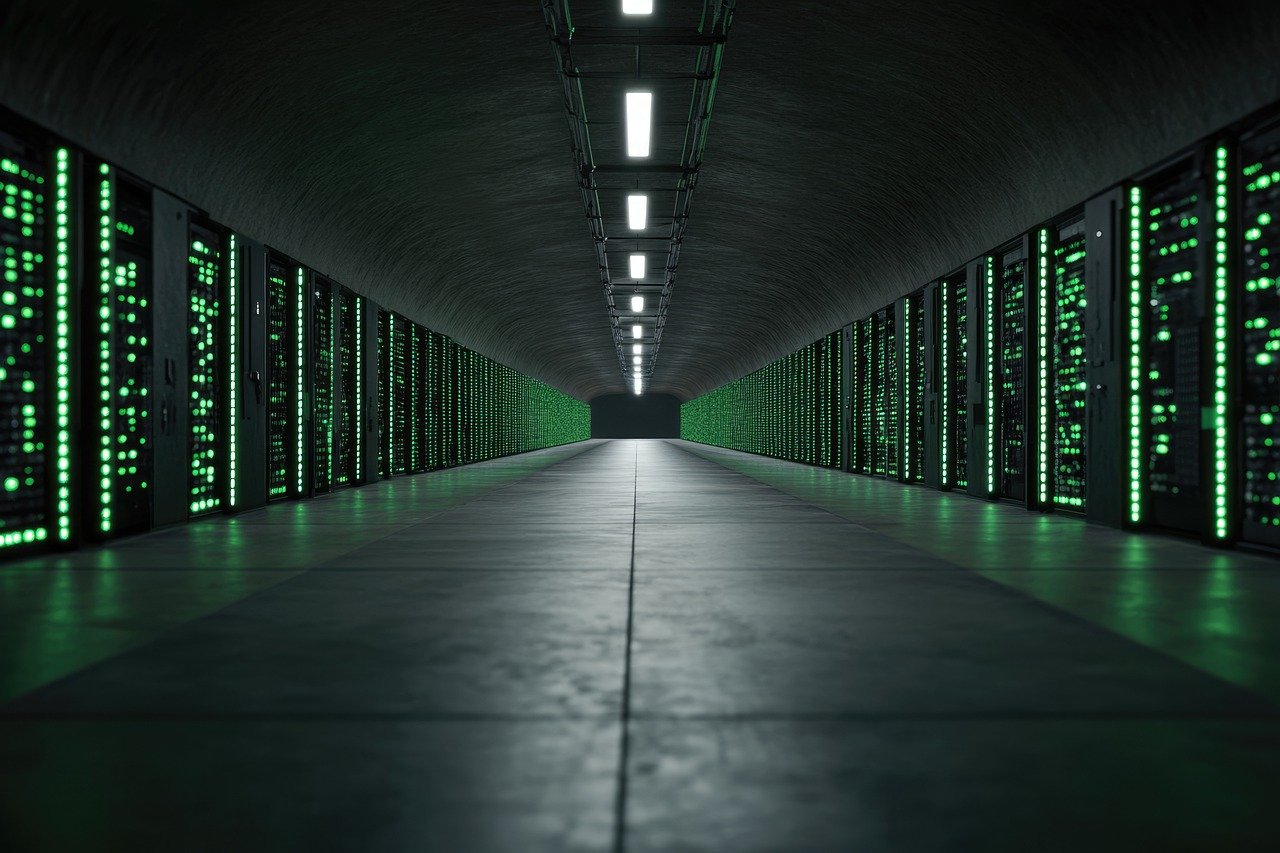
The Demand For Data Centers
Do you know how much energy a ChatGPT query consumes? If you use a traditional Google search to find the answer, that particular Google search would use about 0.0003 kWh of energy, which is enough power to light up a 60-watt light bulb for 17 seconds. A ChatGPT query (or even Google’s own AI-powered search) consumes an estimated 2.9 Wh of energy, which is ten times the energy used by a traditional Google search. Multiply the energy consumed by 200 million ChatGPT daily queries by a year and you have enough power for approximately 21,602 U.S. homes annually or to run an entire country like Finland or Belgium for a day.
No surprise then that AI’s growing mainstream popularity and usage have led to an increase in data center demand. For years, data centers were able to maintain a stable amount of power consumption despite their workloads being tripled, thanks to efficient use of the power they consume. However, that efficiency is now challenged by the AI revolution, with Goldman Sachs Research estimating data center power demand growing 160% by 2030.
McKinsey & Company noted in a September 2024 report that data centers consume 3% to 4% of total US power demand today, while Goldman Sachs puts worldwide use at 1% to 2% of overall power. By the end of the decade, data centers could be seen accounting for 12% of total US power and 3% to 4% of overall global energy. This level of demand spurred investors to push $39.6 billion into data center development and related assets in 2024, which is 12 times the amount spent in 2016.
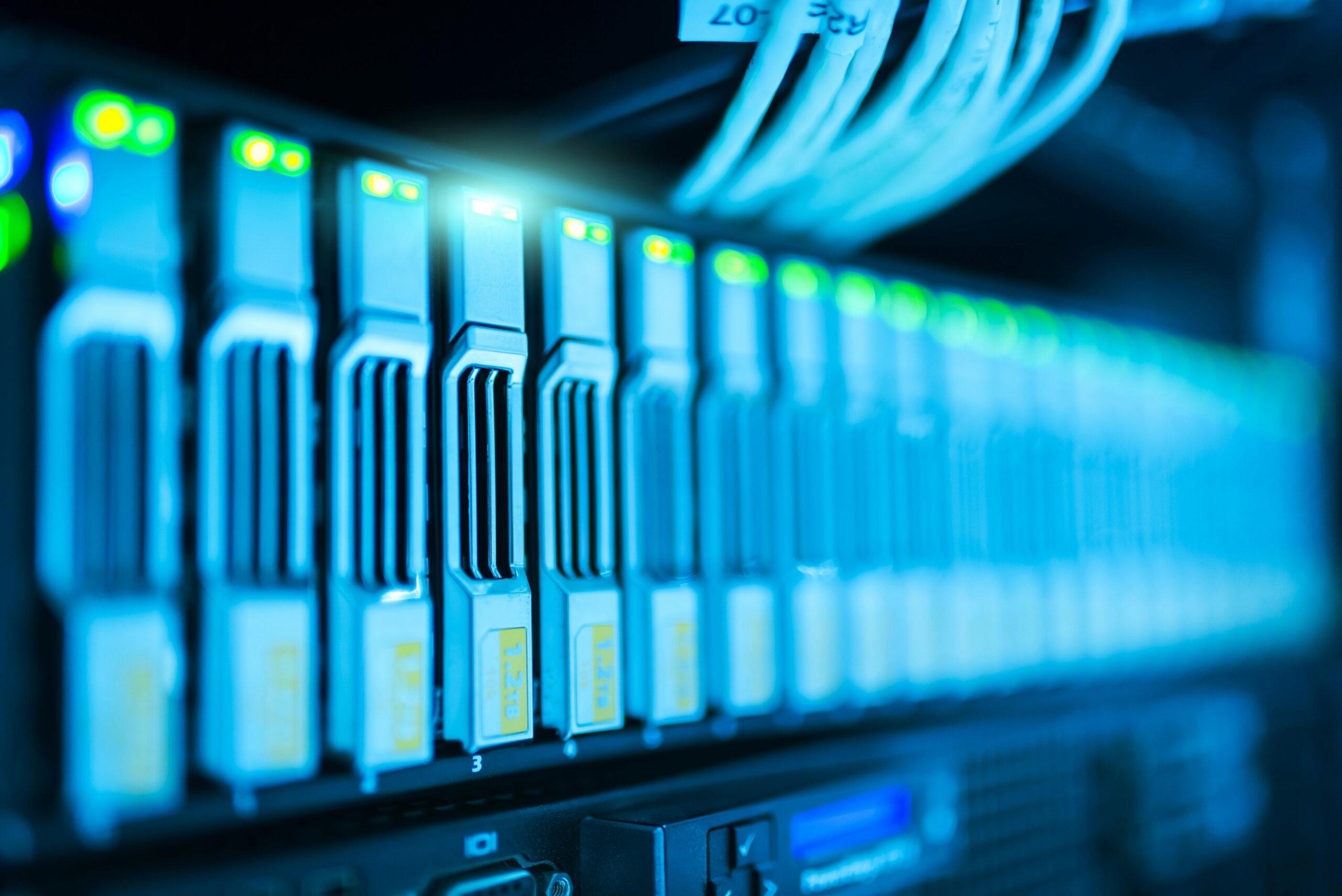
Copyright: panumas nikhomkhai
How All That Power Is Used by AI
What were once warehouse facilities hosting servers mostly found in industrial parks or remote areas, data centers have now progressed into vital institutions in the digital world we’re living in today. The “old” problem used to be that the traditional data centers had some space that was underutilized, while the “new” problem is that space is scarce and critically needed. This has created a surging demand for more of these structures in order to address AI’s accelerating demand for more computing power.
As illustrated earlier, AI workloads consume more power than traditional counterparts like cloud service providers. Out of the two primary AI operations, “training” requires more computing power to build and expand models over time. However, the operation that derives responses from existing models called “inferencing” is growing quickly in volume as AI-powered applications become more popular and widely adopted according to a Moody’s Rating report. Their report further predicts inferencing growth over the next five years to make up a majority of AI workloads.
Amazon, Google, Microsoft, and Meta have stepped up to satisfy the demand by building, leasing and developing plans for hyperscale data centers. Moreover, investors have demonstrated a pronounced prioritization of AI projects over traditional IT investments; in fact, an Axios article noted that “tech leaders are actually worrying about spending too little.”
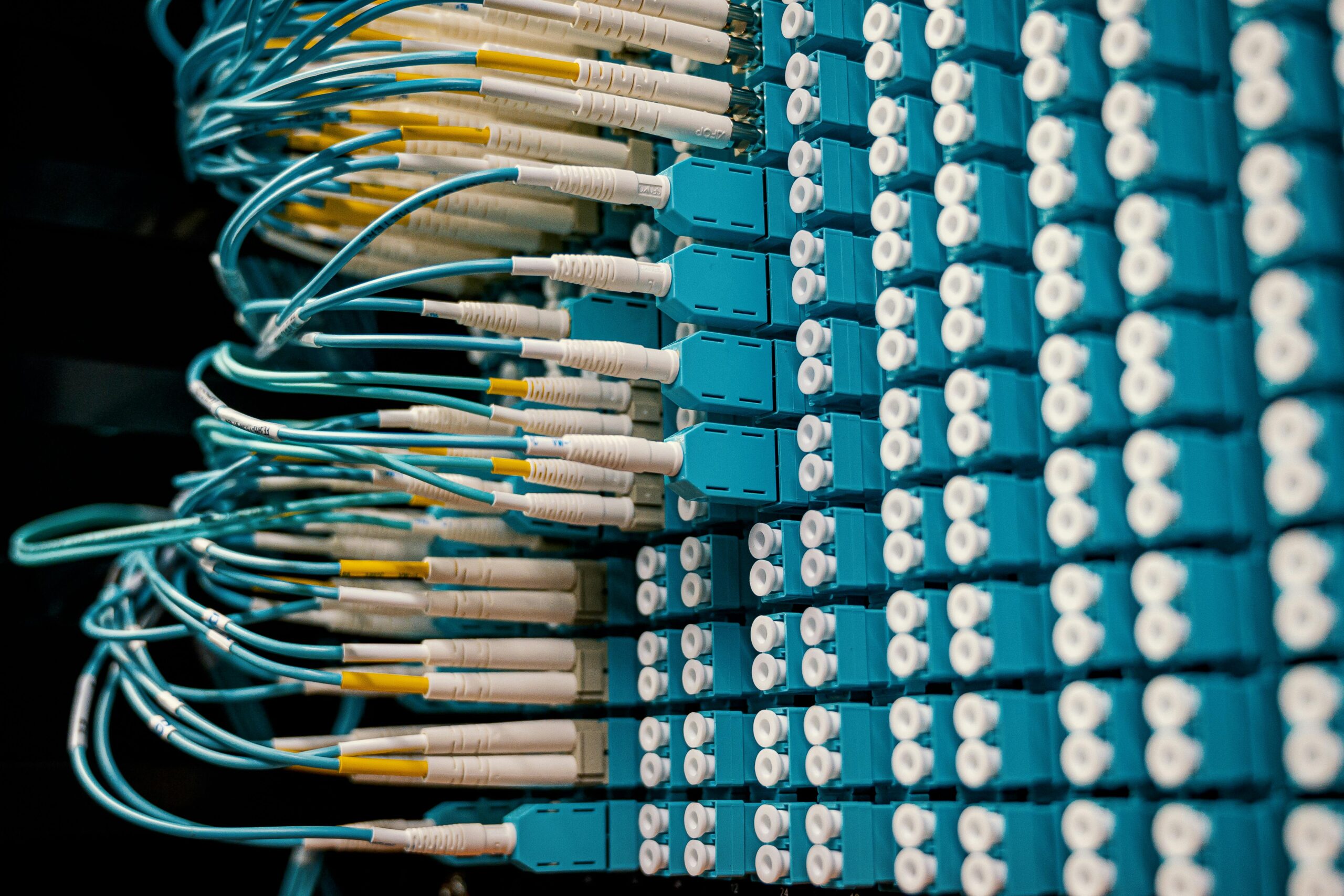
Copyright: Brett Sayles
Chokepoints in the AI Boom
It’s not only power that tech companies and developers have to consider when addressing the demand for new data centers; their location and access to that power are challenging their progress and development. Data centers may consume up to 4% of US power today, but because they are clustered in certain major markets they pose a substantial stress to local resources. McKinsey & Company’s September 2024 report also noted that there is at least a three-year wait time for new data centers to tap into the power grid of a major market like Northern Virginia. The search for available space, power and tax incentives have led developers to look into other markets like Dallas or Atlanta, according to a Pitchbook article.
Unlike investors, some local governments are not as keen on the development of data centers and may pause contracts or prioritize granting access to their power grid to other projects. An October report by the Washington Post revealed that a number of small towns have strongly pushed back against the construction of data centers in their areas.
In addition to concerns from residents over the strain data centers would put on local resources, carbon dioxide emissions can’t be overlooked, as Goldman Sachs estimates that such emissions will more than double by 2030. Areas with rising energy consumption from data centers might therefore find it challenging to meet climate targets.
Accelerated data center equipment demands are also straining the supply chain, as orders are taking years to fulfill. Planned tariff increases could also stress the delivery of offshore-produced parts and components used by data centers.
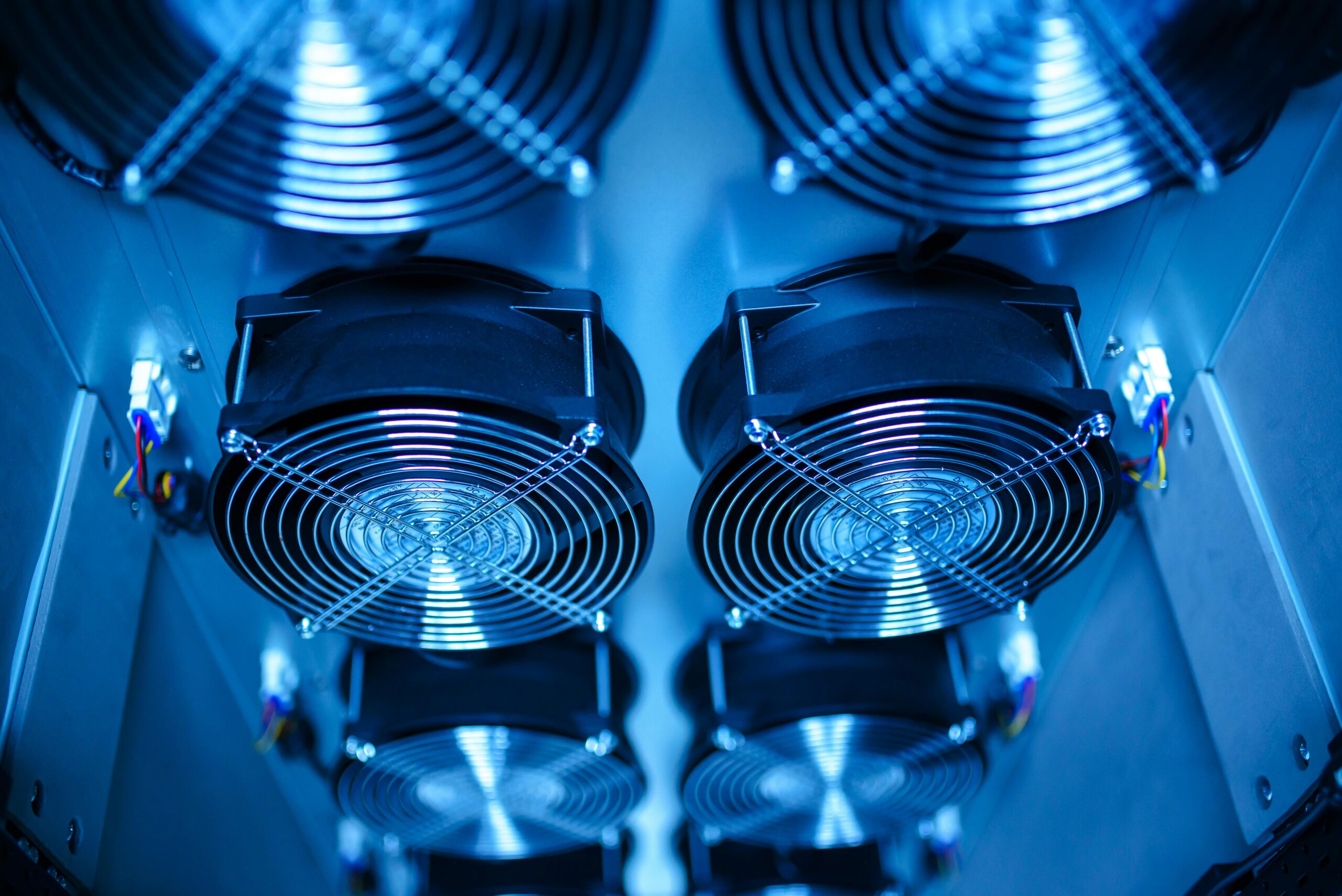
Copyright: panumas nikhomkhai
Navigating Towards The Future
While it is exciting to witness AI’s mainstream adoption and technological advancements, the challenges brought forth by its explosive demand for data centers could be just as intimidating. Perhaps Forbes expressed best how we should navigate this period of growth and uncertainty: we need to plan with purpose, not panic. We need to build with responsibility, not exuberance.
While the AI boom granted us the opportunity to correct the oversupply of demand centers resulting from the dot-com boom, we need to tread more carefully when addressing this new need for more of these infrastructures. We need to understand what each data center is being planned and built for, its primary purpose, and how it connects to local resources. Yes, we were able to breathe new life into data centers that were underutilized after the Internet boom, but that took years and a new technological revolution to correct. Who knows if we’re also able to enjoy the same chance to recover if we fumble today’s attempts to address the demand for data centers? For all we know, the next technological wave might such be a swerve that it advances beyond the need for data centers.
We should also improve, innovate or discover new renewable and responsible energy solutions as well as increase the efficiency of our developing technologies’ use of power. There’s also an opportunity for the tech industry to enter into dialogues with local audiences about what is happening in the digital world and what they can expect from AI, thus demonstrating transparency and social responsibility.
As investors and developers eagerly meet AI’s surging demand for data centers, perhaps we can pause and appreciate this opportunity to make decisions that not only fortify the connection between the digital space and the world we live in today, but also forge a more responsible and sustainable path towards the future.
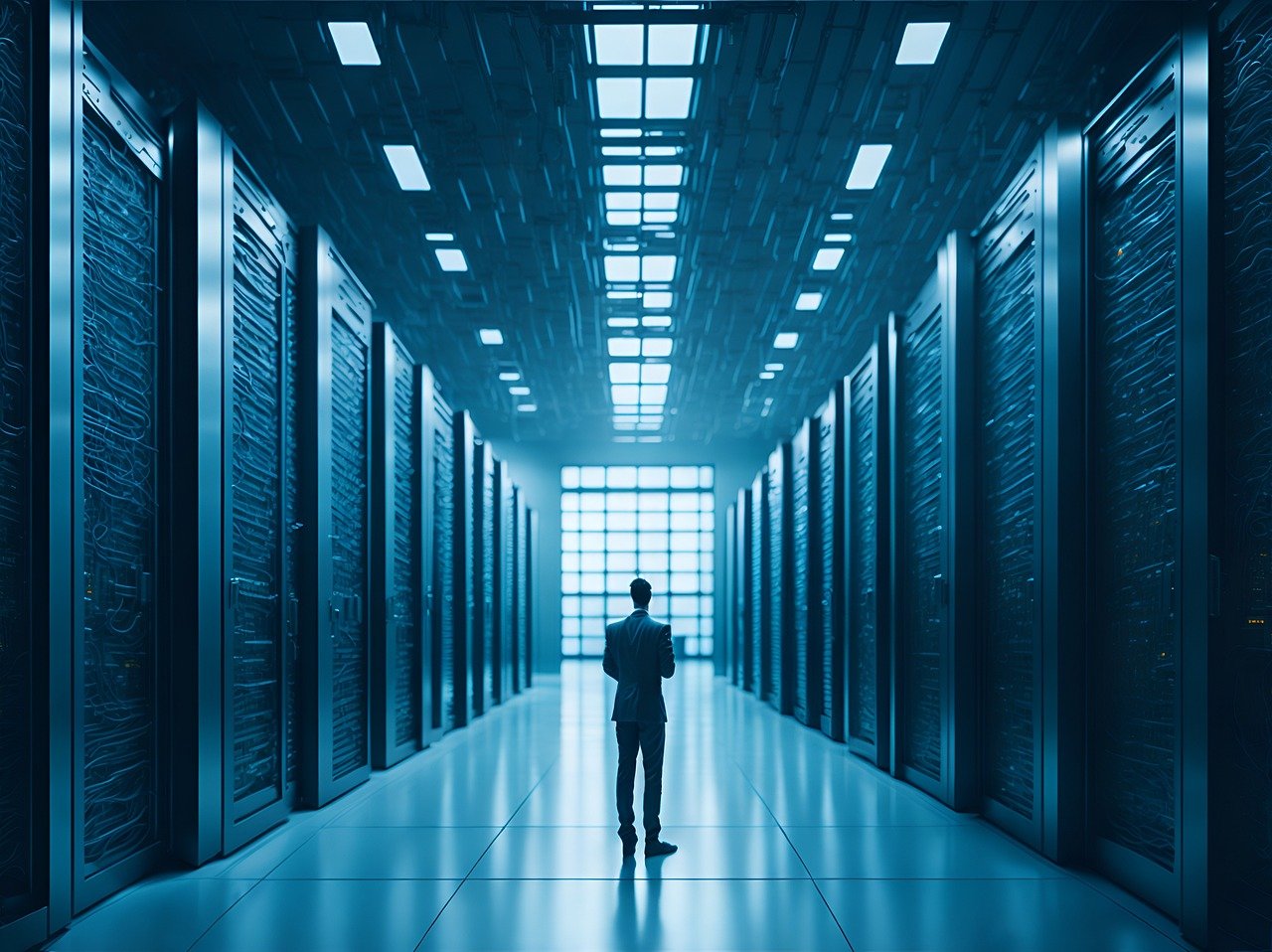
Copyright: TheDigitalArtist
The Impact of DeepSeek
While the tech industry searches for solutions to the data center demand, a new player has emerged to shake things up: Chinese AI research lab DeepSeek has released their open-source large language model. Quickly shooting to the top of Apple Store’s downloads, DeepSeek has challenged contemporary views on AI development with a platform that performs just as powerfully and efficiently as OpenAI while reportedly operating at a fraction of its Western counterparts’ cost. While it feels like we’re on the brink of a paradigm shift, we believe its true impact is yet to be seen. We expect to know and learn more in the coming weeks, and we’ll share our thoughts in a future blog.
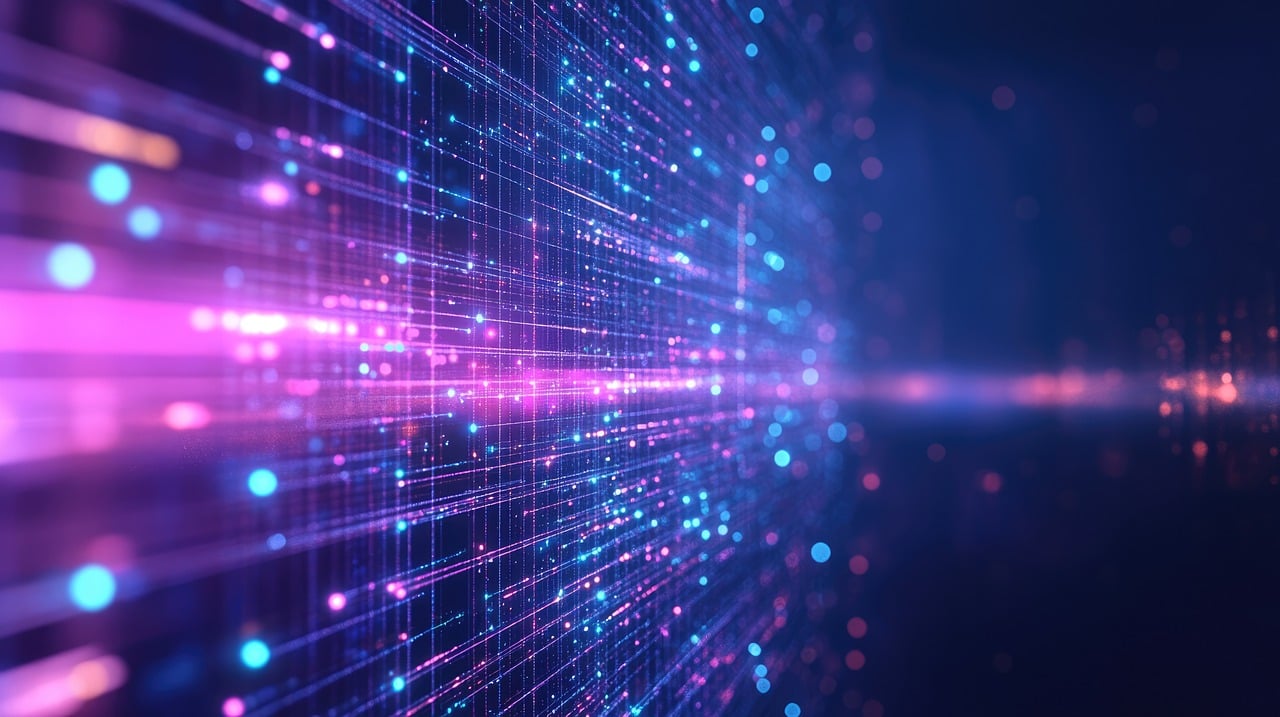
Copyright: Yanu_jay
Featured Image Copyright: franganillo
Top Image Copyright: Yamu_Jay
Read more